Accelerating materials-space exploration for thermal insulators by mapping materials properties via artificial intelligence
npj Computational Materials(2023)
摘要
Reliable artificial-intelligence models have the potential to accelerate the discovery of materials with optimal properties for various applications, including superconductivity, catalysis, and thermoelectricity. Advancements in this field are often hindered by the scarcity and quality of available data and the significant effort required to acquire new data. For such applications, reliable surrogate models that help guide materials space exploration using easily accessible materials properties are urgently needed. Here, we present a general, data-driven framework that provides quantitative predictions as well as qualitative rules for steering data creation for all datasets via a combination of symbolic regression and sensitivity analysis. We demonstrate the power of the framework by generating an accurate analytic model for the lattice thermal conductivity using only 75 experimentally measured values. By extracting the most influential material properties from this model, we are then able to hierarchically screen 732 materials and find 80 ultra-insulating materials.
更多查看译文
关键词
thermal insulators,mapping materials-space properties,exploration
AI 理解论文
溯源树
样例
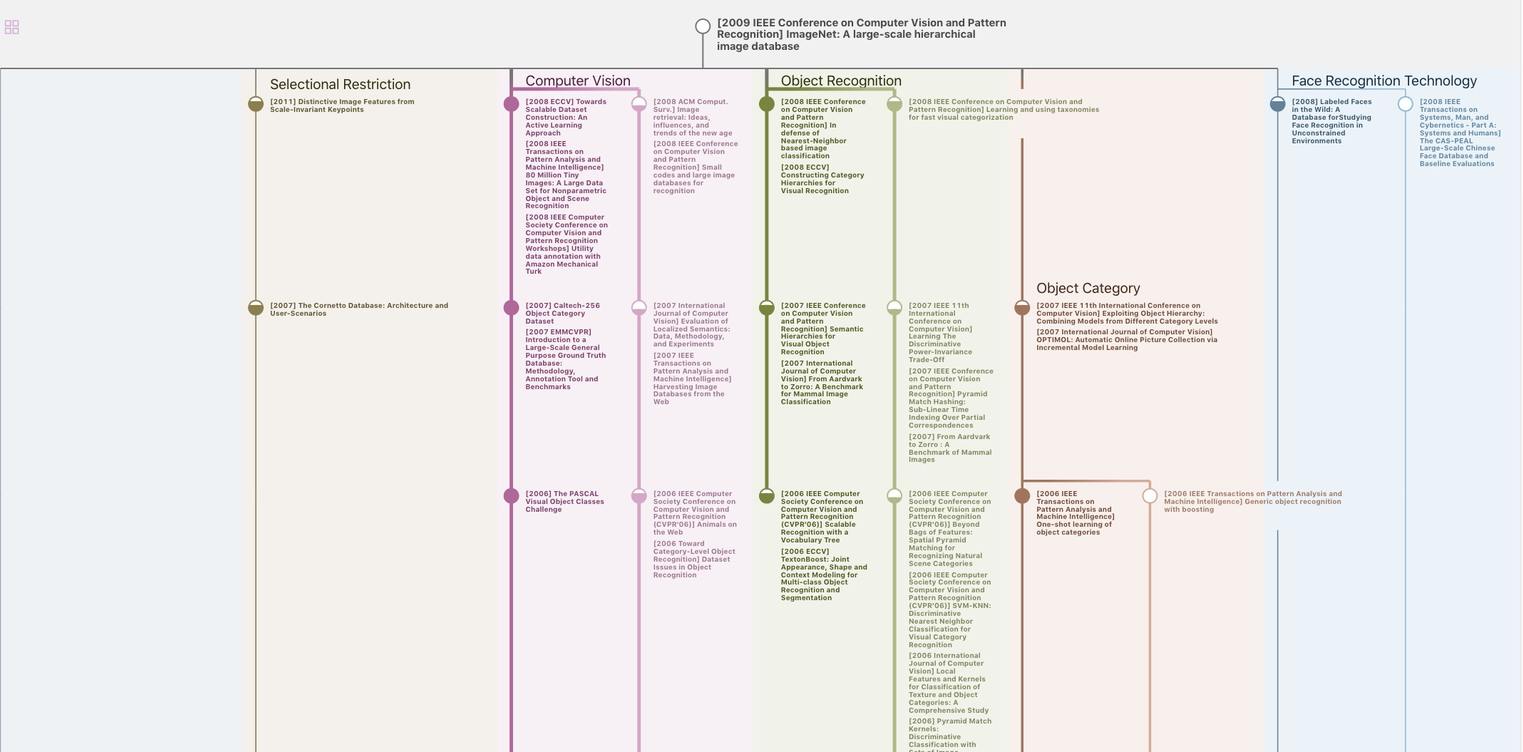
生成溯源树,研究论文发展脉络
Chat Paper
正在生成论文摘要