A machine learning approach for accelerated design of magnesium alloys. Part A: Alloy data and property space
JOURNAL OF MAGNESIUM AND ALLOYS(2023)
摘要
Typically, magnesium alloys have been designed using a so-called hill-climbing approach, with rather incremental advances over the past century. Iterative and incremental alloy design is slow and expensive, but more importantly it does not harness all the data that exists in the field. In this work, a new approach is proposed that utilises data science and provides a detailed understanding of the data that exists in the field of Mg-alloy design to date. In this approach, first a consolidated alloy database that incorporates 916 datapoints was developed from the literature and experimental work. To analyse the characteristics of the database, alloying and thermomechanical processing effects on mechanical properties were explored via composition-process-property matrices. An unsupervised machine learning (ML) method of clustering was also implemented, using unlabelled data, with the aim of revealing potentially useful information for an alloy representation space of low dimensionality. In addition, the alloy database was correlated to thermodynamically stable secondary phases to further understand the relationships between microstructure and mechanical properties. This work not only introduces an invaluable open-source database, but it also provides, for the first-time data, insights that enable future accelerated digital Mg-alloy design. (c) 2023 Chongqing University. Publishing services provided by Elsevier B.V. on behalf of KeAi Communications Co. Ltd. This is an open access article under the CC BY-NC-ND license ( http://creativecommons.org/licenses/by-nc-nd/4.0/ ) Peer review under responsibility of Chongqing University
更多查看译文
关键词
Magnesium,Alloy design,Mg-alloy database,Data analysis,Data visualisation,Unsupervised machine learning.
AI 理解论文
溯源树
样例
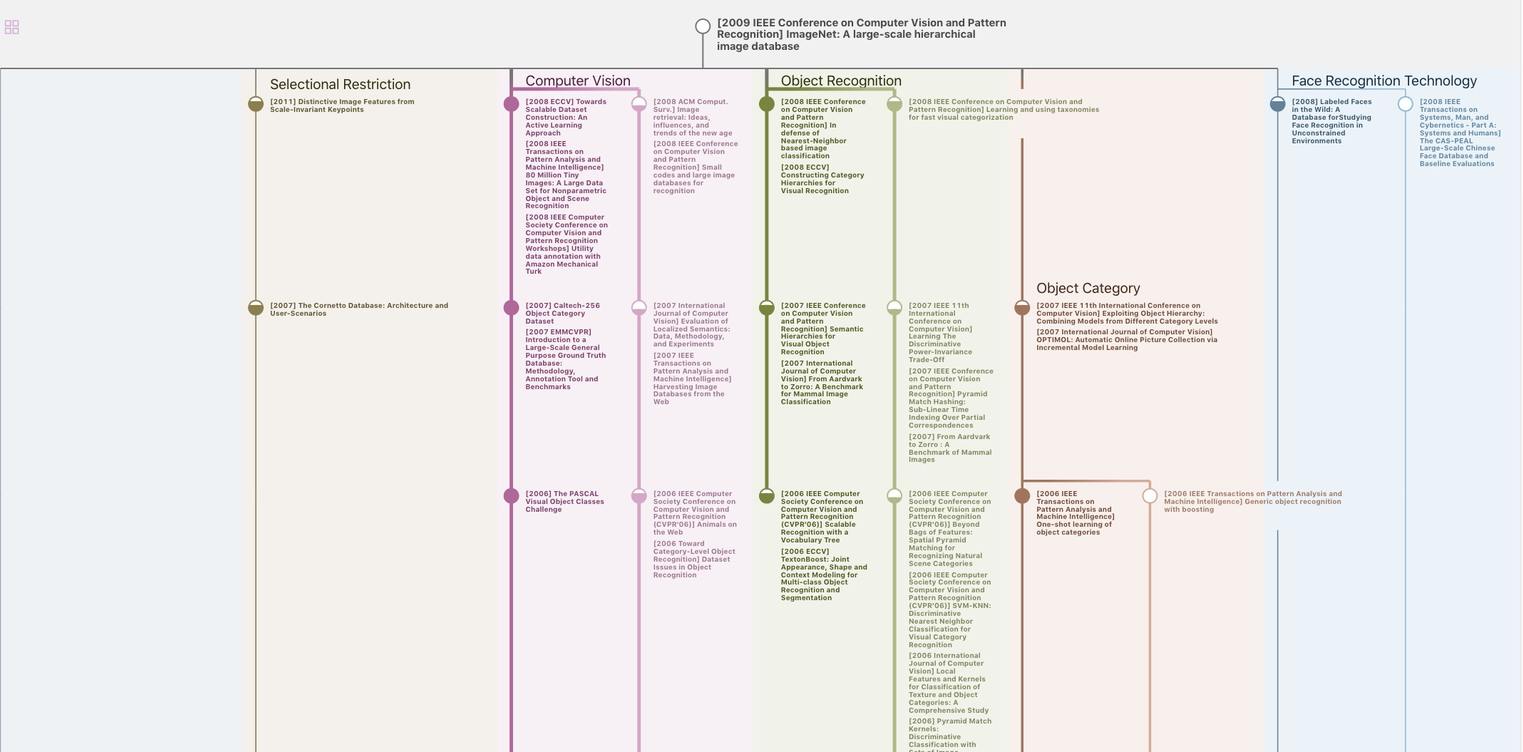
生成溯源树,研究论文发展脉络
Chat Paper
正在生成论文摘要